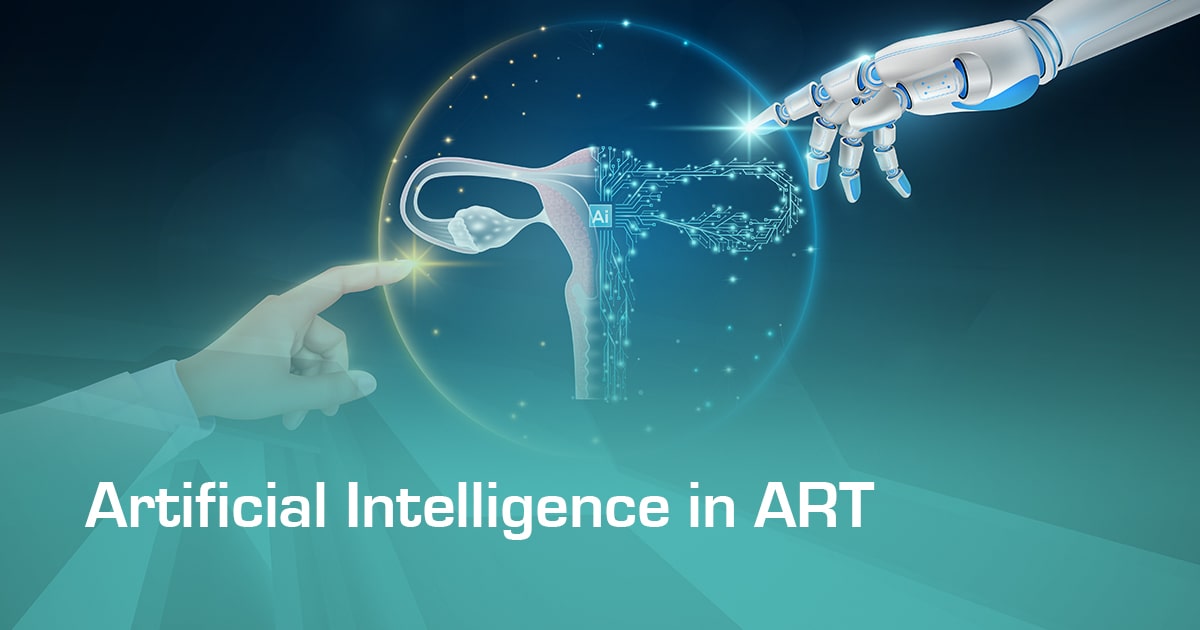
John McCarthy first introduced the term "AI" in 1955 during the Dartmouth Summer Research Project on Artificial Intelligence. Over four decades ago, the first successful in vitro fertilisation (IVF) procedure resulted in the birth of a baby. Since then, IVF techniques have seen significant advancements, including tailored ovarian stimulation, extended embryo culture at physiological oxygen levels, and a shift towards single embryo transfer.
Over the past four decades since the first successful IVF, there has been remarkable advancement in Assisted Reproductive Technologies (ART). This includes techniques like oocyte and embryo cryopreservation, assisted fertilization, preimplantation genetic testing, and embryo selection, all of which have significantly improved clinical pregnancy rates. Nevertheless, challenges persist, particularly in accurately assessing the quality of eggs, sperm, and embryos. Although personalized approaches have improved outcomes, roughly only one-third of all IVF cycles result in pregnancy on average. Addressing this issue, AI holds the promise of enhancing IVF cycle efficiency, potentially reducing failed retrievals, transfers, and miscarriages, ultimately leading to healthier live births from single, euploid embryos.
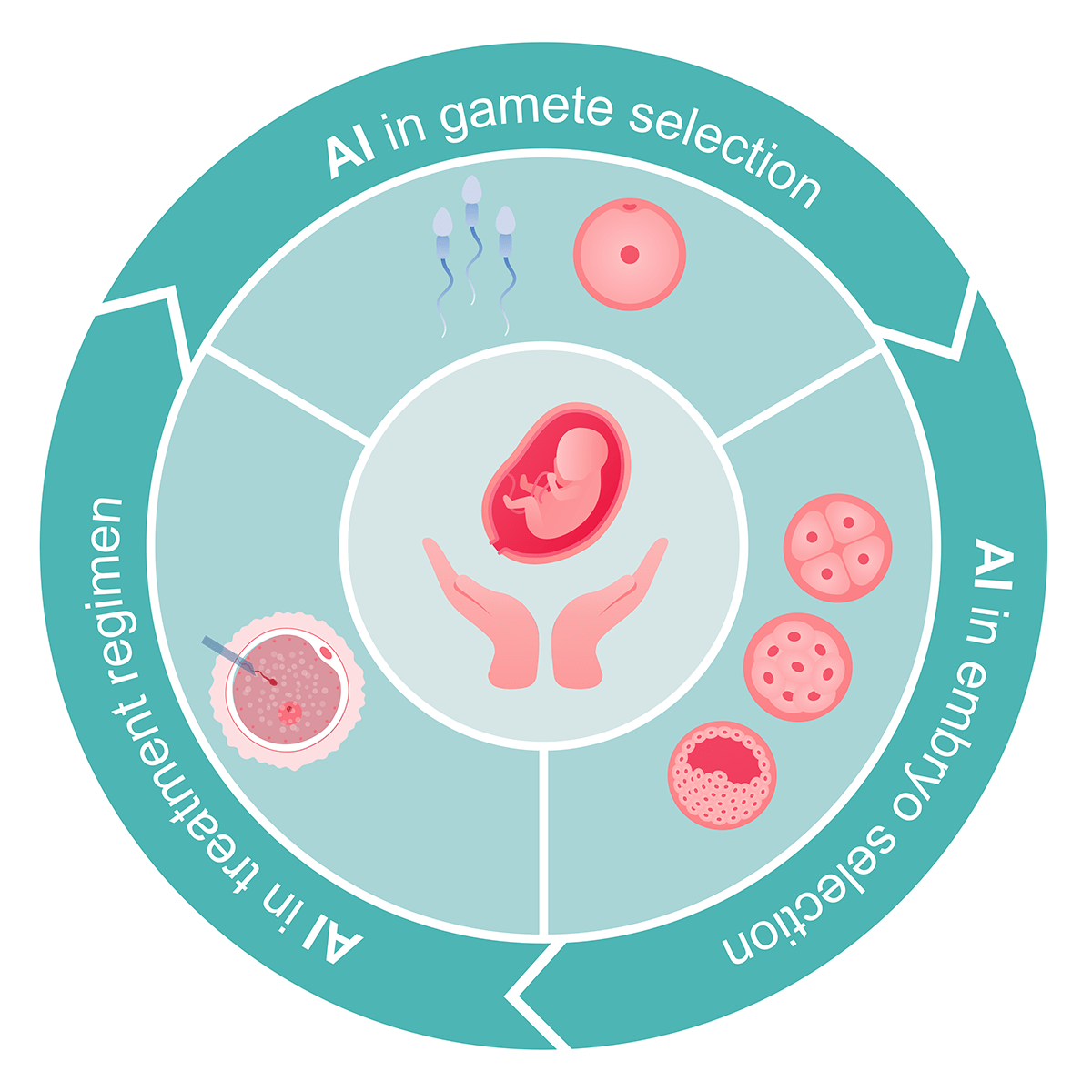
Role of AI in ART:
AI is poised to play a pivotal role in various aspects of the IVF process. Recent literature emphasizes its potential in gamete and embryo selection, as well as in tailoring treatment regimens for improved IVF outcomes.
AI's primary applications in this field include ongoing research to identify reliable non-invasive markers that can bolster implantation rates and enhance ART effectiveness. Integrating AI with image analysis can enhance recognition accuracy, minimize errors, and reduce the manual workload associated with classifying sperm, embryos, and oocytes.
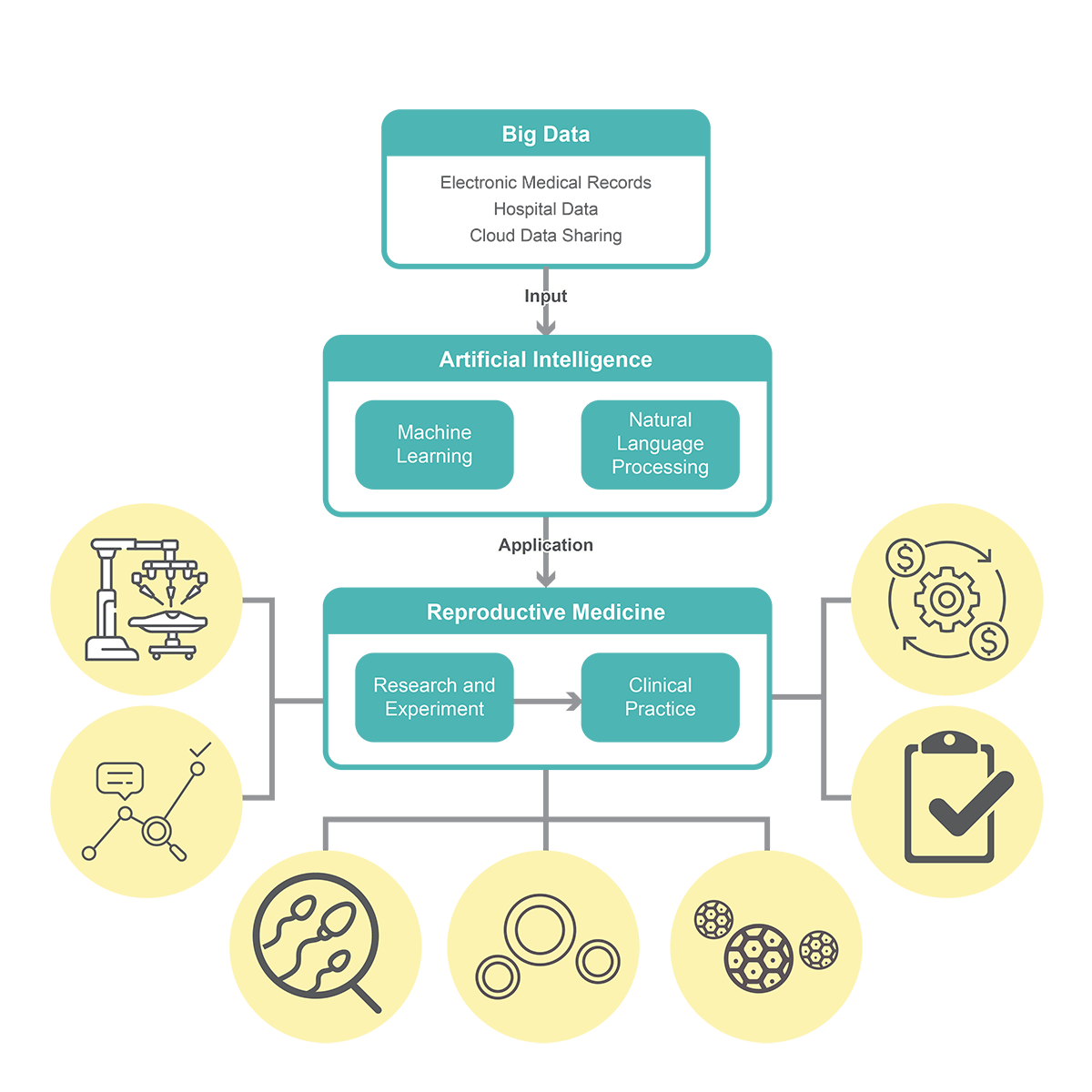
How does AI help in monitoring oocytes?
The overall reproductive success, whether through natural means or assisted reproductive techniques (ART), hinges significantly on the quality of oocytes. Presently, the pregnancy rate per retrieved oocyte stands at approximately 4.5%. A deeper comprehension of oocyte developmental competence holds the potential to shape novel strategies for enhancing IVF success rates, along with identifying new biomarkers for predicting oocyte quality and selecting the most suitable egg for IVF.
Various methodologies have been suggested for appraising and picking out oocytes with the highest developmental potential. However, there are inherent limitations, such as the likelihood that seemingly normal oocytes or embryos may still harbor aneuploidy, necessitating further investigation to establish precise criteria and techniques. Thus, the integration of AI techniques for oocyte selection within IVF programs presents a promising avenue. An observational study was conducted which tracked the maturation process of mouse oocytes from the germinal vesicle (GV) to the metaphase II stage and recorded images for later time-lapse analysis. Utilizing particle image velocimetry (PIV) to analyse cytoplasmic movement velocities, they employed a feed-forward artificial neural network to differentiate between competent and incompetent oocytes with an accuracy of 91.03%.
The optimal approach to oocyte selection would ideally be non-invasive, cost-effective, and seamlessly integrated into embryology workflows with minimal disruption. ART procedures still harbour room for refinement, particularly in technologies that offer more dependable predictions of oocyte quality and more precise quantification of gamete developmental competence. Moreover, the application of AI techniques to the assessment of human oocytes, incorporating time-lapse methods or scrutinizing gene expression through transcriptomics or genomics holds significant potential for further advancing ART practices.
How does AI help in sperm selection?
Semen analysis constitutes the initial step in assessing infertility among couples. Sperm morphology serves as an indicator of irregularities in human semen samples. The ability to accurately discern the morphology of sperm cells and track changes in their motility is crucial for gauging sample fertility potential. At present, computer-aided sperm analysis (CASA) systems are utilized in both human and animal research. They furnish data on motility percentages, kinematic parameters, and enable the identification of distinct subpopulations of sperm cells. (Goodson et al. 2017).
Given the inherent subjectivity and challenges associated with manually evaluating sperm morphology, coupled with considerable variability across laboratories, it is imperative to develop automated image analysis-based methods to yield more precise and objective results. Additionally, a significant portion of male factor infertility, up to one-third, remains idiopathic, indicating that current sperm assessment methods may not capture all potential causes of infertility (Gudeloglu et al. 2014).
In 2011, Goodson and colleagues presented a quantitative, automated method for classifying the motility patterns of mouse sperm. This approach relied on data gathered from the CASA system, involving analysis of 2043 sperm tracks. This method was subsequently applied to human sperm in 2017 (Goodson et al. 2017), achieving an overall accuracy of 89.92%. The model was retrospectively validated using data from 425 human sperm samples, enabling the diagnosis of chromosomal abnormalities. Significantly, attributes including height, total testicular volume, levels of follicle-stimulating hormone, luteinizing hormone, total testosterone, and ejaculate volume were considered. This comprehensive approach achieved a prediction accuracy of over 95% for chromosomal abnormalities.
How does AI help in embryo selection?
Accurate evaluation of embryo viability is crucial for optimizing IVF treatments and increasing pregnancy rates. Usually, embryologists depend on non-invasive visual evaluations of embryos or oocytes. This assessment emphasizes characteristics like morphology and dynamic development, particularly during the blastocyst stage. However, this evaluation is inherently subjective, leading to variability between different observers and even within the same observer, influenced by factors such as embryo scoring systems and the embryologists experience and expertise, ultimately affecting the final success rate. Moreover, the transfer of multiple embryos per cycle, while potentially increasing the success rate, raises the risk of complications like pre-eclampsia and maternal haemorrhage, as well as the likelihood of multiple pregnancies.
The integration of AI with automated morphological analysis of embryos or blastocysts presents an enticing solution. In 2012, Santos Filho and colleagues presented a semi-automated grading technique. This method involved tasks such as image segmentation and classification specifically applied to human blastocyst images. The researchers employed SVM classifiers to evaluate the inner cell mass (ICM) and trophectoderm (TE) quality. Through an analysis of fractal dimensions and mean thickness in TE and ICM image textures, they successfully characterized the crucial morphological attributes of the blastocyst. Additionally, optimizing microscope settings, such as enhancing contrast and delineating individual features more distinctly, may further refine image analysis.
Singh et al. (2015) proposed an innovative algorithm for the automatic identification of TE regions in human blastocysts. Leveraging the Retinex algorithm to enhance input image quality, they achieved an impressive average shape accuracy of 87.8% for TE region detection.
In 2017, Saeedi and co-authors introduced the first automated approach for concurrently segmenting both the trophectoderm (TE) and inner cell mass (ICM) in images of human blastocysts. Through the creation and testing of a diverse dataset comprising 211 blastocyst images of varying grades, they reported accuracy rates of 86.6% for TE identification and 91.3% for ICM identification. Despite these advances, embryo morphology remains the primary tool for selection and transfer. The data derived from automatic image identification offers a valuable opportunity for objective embryo assessment and enables a more quantitative analysis.
Methods reliant on morphokinetic parameters for embryo selection have been published. Furthermore, the Time-Lapse (TL) dynamic monitoring system offers continuous data regarding embryo development and morphokinetics. In 2017, Carrasco and colleagues performed a retrospective examination of 800 human embryos with documented implantation data, employing a Time-Lapse system. They developed a model by scrutinizing morphokinetic parameters and evaluating embryo morphology on Day 3. The assessment of morphokinetics played a pivotal role in the exclusion of embryos with the lowest potential for implantation.
Conclusion:
Incorporating AI into fertility clinics holds the promise of significantly enhancing IVF success rates. It could offer swift, objective, and accurate assessments of gamete and embryo health, supporting embryologists. Moreover, AI has the potential to aid physicians in customizing fertility treatment strategies according to the unique characteristics of each patient.
An AI-powered ART software offers numerous advantages, including reducing interobserver variability, fine-tuning drug doses in oocyte stimulation to mitigate adverse effects like hyperstimulation, minimizing in-person medical interactions to enhance overall productivity for both healthcare providers and users. Additionally, it improves the selection of sperm samples and enhances the assessment of oocyte quality and embryo selection.
However, several considerations arise when implementing AI in ART. These encompass safeguarding personal data in compliance with pertinent legislation and incorporating human expertise into clinical decision-making processes. Overcoming these challenges, an algorithm encompassing the variables discussed in the scope of this topic holds the potential for direct clinical application, likely becoming an integral tool adopted by leading fertility clinics worldwide.
References:
1. JM Raimundo;P Cabrita; (2021). Artificial intelligence at assisted reproductive technology . Procedia Computer Science, (), –. doi:10.1016/j.procs.2021.01.189